The STIHL Group has been a global name in the development, manufacturing, and distribution of power tools for forestry and agriculture since 1926. Servicing professional forestry and agricultural sectors as well as construction and consumer markets, STIHL has been the world’s top-selling chainsaw brand since 1971.
With a worldwide sales volume of nearly 4 billion euros1 and a workforce of 16,722, STIHL oversees its own manufacturing plants in seven countries—Germany, USA, Brazil, Switzerland, Austria, China, and the Philippines. Maintaining a high degree of manufacturing verticality ensures key knowledge is developed and upheld in-house.
Quality assurance is integral to STIHL’s production process; as part of an upgrade, STIHL sought a fully automated solution for visual quality assessment. Previously, “the objective visual quality test was carried out by people,” says Alexander Fromm, engineer for automation systems at STIHL Group. “The test and success of a vision system was that the same assessment can be made using neural network technology at the very least.”
From Human Eyes to Machine Learning
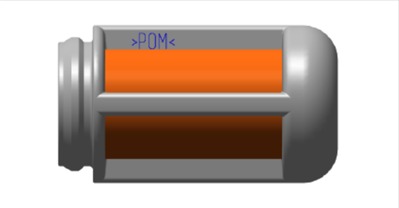
The inspection that STIHL sought to enhance focuses on the production of gasoline suction heads as a component of a chainsaw. Filtering dirt, wood shavings, and other invasive elements, these suction heads are integral to ensure that no dirt particles enter the combustion chamber, which could cause damage to the power tool.
The gasoline suction heads consist of a plastic body and a piece of fabric which is applied later in the assembly. These suction heads are a semi-finished product at this point in their manufacturing, and the inspection occurs midway through the production process. Each suction head features four footbridges that must be assessed independently after the injection molding, and before the part proceeds to the next step in the manufacturing process.
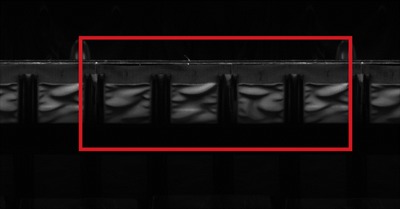
It is crucial to assess and classify the seams on these components to ensure that the footbridges are adequately positioned and sealed before they are used. The footbridge gives the component its stability, stretching the fabric of the filter and enclosing the fabric seam so it does not tear open.
Prior to implementation of STIHL’s new system, human operators would perform an objective visual quality test to determine whether the components were adequate. Though the production machine was always automated, operator intervention was necessary when the output started to deviate from the high quality standards at STIHL. In those cases, the operator would need to visually inspect the batch of parts to determine if the production machine had become problematic.
STIHL sought a new solution, one that would replace the human element with machine vision based on deep learning. The quality-assurance assessment would thus be automated to cut costs and save time. “When we began the process of considering a machine vision solution, every gasoline suction head was being checked by a human,” says Fromm, “however, the parts are very small and the error features are quite hard to detect, so we determined a need for deploying machine vision into the inspection process.” Slip rates are defined as instances wherein a bad part is erroneously classified as a good part; hit rates are accuracy results.
“We have been working with Matrox Imaging since 2016,” Fromm continues, “when STIHL forged a relationship with Rauscher GmbH—a key Matrox Imaging and machine vision component provider in Germany – after meeting at a trade fair. We appreciate being able to work with a single supplier to get both hardware and software from one vendor, as that has been instrumental in getting our systems up and running quickly. It’s because of our positive history with Rauscher GmbH that STIHL sought the expertise of Matrox Imaging from development of this new system.”
Going Deeper with Deep Learning
“The inspection process of each part involves looking at four distinct footbridges, and the machine processes 60 parts per minute. The inspection therefore occurs at a rate of 240 images per minute,” Fromm outlines. Conventional image processing tools had been used to evaluate the parts; deep learning functionality extends the field of image processing capabilities in instances where conventional image processing produces inconclusive results, due to high natural variability. “STIHL determined that rule-based image processing is not appropriate, because the component images vary too much and the error rate is too high, even at hit rates ranging from 80% to 95%,” Fromm concludes. “The new system would thus be required to yield fewer slips and result in a higher hit rate. Using Matrox Imaging’s classification steps yielded hit rates of 99.5% accuracy, a tremendous improvement.”
STIHL’s new vision system comprises Matrox Design Assistant X vision software running on a Matrox 4Sight GPm vision controller, selected because of the I/O capabilities, PROFINET connections, and Power-over-Ethernet (PoE) support. The system also includes a PoE line-scan camera, a rotary table, an encoder, and ultra-high intensity line lights (LL230 Series) from Advanced illumination.
Development and deployment of STIHL’s new vision system brought together vision experts from STIHL’s team, members of Rauscher GmbH’s applications team, as well as several machine-vision experts from the Matrox Vision Squad.
Good, not good, and where the differences lie
Effective training of a neural network is not a trivial task; images must be adequate in number, appropriately labeled, and represent the expected application variations on a set-up that yields repeatable imaging conditions. With this in mind, the team at STIHL engaged Matrox Imaging’s vision experts to undertake the training of the convolutional neural network (CNN) on their behalf.
Fromm describes the collection of images as representing “a plastic part with fabric seam, photographed from the inside. In the images, only the footbridge itself contains important information, everything else is irrelevant. To prepare the dataset, therefore, each footbridge is extricated from the general image and sorted into folders classed as ‘good (IO)’ and ‘not good (NIO)’. The team at STIHL undertook the process of manually labeling 2,000 representative parts, each with four images, for a total dataset of 8,000 images. Without the guidance of Matrox Imaging’s engineering team, this level of intricacy would have been exceptionally challenging.”
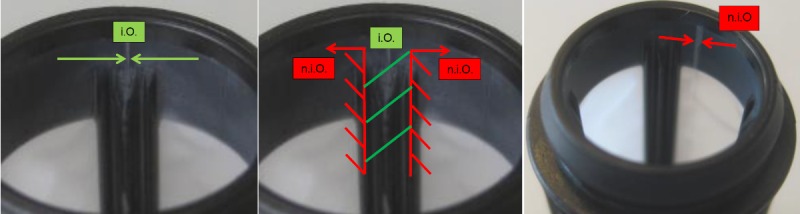
The collection of 8,000 images was provided to Matrox Imaging’s team of vision experts, who employed MIL CoPilot interactive environment to train the CNN and produce a classification context file, which was subsequently returned to STIHL for importing into Matrox Design Assistant X software environment and used to automatically classify new images into these pre-determined classes. MIL CoPilot gives access to pre-defined CNN architectures and provides a user-friendly experience for building the image dataset necessary for training.
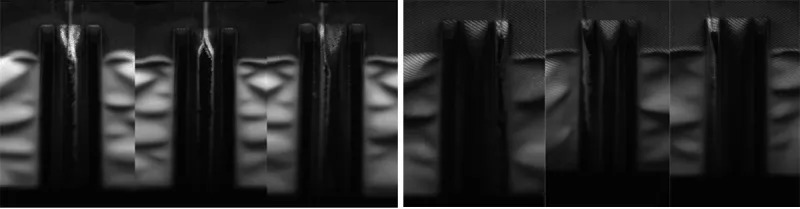
Fromm affirms that “our main point of contact was through Rauscher GmbH; we received quick answers and very good support. As the system was being brought online, the STIHL team undertook online training using the Matrox Vision Academy portal to help strengthen our knowledge of how best to use the Matrox Design Assistant X machine vision software.”
Bringing it Online
With support from Matrox Imaging, STIHL successfully navigated the challenge of establishing a correct and repeatable presentation of the footbridge to facilitate taking images for training the CNN. Another challenge was simply collecting the sheer volume of images necessary, as well as the careful cutting, sorting, and labelling of the images. “It was a challenge,” Fromm notes, “but the more time you invest in procuring good images, the better results you get!”
Conclusion
Their new vision system now deployed, STIHL is overwhelmingly pleased with the enhancements that Matrox Design Assistant X’s deep-learning-based classification tools have made to their quality-assurance measures. Plans are already underway to develop a second, similar system; image collection and CNN training has already begun.
“Matrox Imaging and Rauscher GmbH are very good partners; STIHL has been using Matrox Imaging software, components, and systems for a number of years,” Fromm concludes. “Deep learning technology extends the field of image processing, in which conventional image processing yields inadequate results. Implementation of this new system—one that effectively deploys deep learning—has replaced objective visual processes that STIHL had in place. As a result, we anticipate great improvements in our efficiency, with the ability to perform new tasks, ensuring an overall improvement in the quality of our products.”
For more information: www.matrox.com
Tags: 3d vina, and Human Error, Deep Learning Cuts Through Cost, hiệu chuẩn, hiệu chuẩn thiết bị, máy đo 2d, máy đo 3d, máy đo cmm, sửa máy đo 2d, sửa máy đo 3d, sửa máy đo cmm, Time