3D Imaging is Everywhere
3D point cloud image capture and RGB-Z color images are becoming indispensable tools for a wide range of applications. From Apple’s FaceID™ to industrial machine vision, in-car infotainment, and situationally aware home robots, a variety of 3D imaging methods are making their way into our devices, our homes, and our workplaces.
Several enabling technologies have been implemented, with some of the better-known solutions using stereo cameras, structured light, and time-of-flight methods, or novel technologies such as DEPTHIQ™ from AIRY3D which takes an economical approach in 3D image sensor design.
Most of the conventional 3D techniques come with a certain degree of complexity and various performance limitations. For example, a common limitation of a stereo camera is that certain parts of the scene are not situated suitably to allow both ‘eyes’ of a stereoscopic camera to apply the binocular vision principle to infer the depth from the disparity between the left and right cameras. Solutions that rely on structured or pseudo-random light projection may be severely challenged by the presence of windows or other hard, shiny surfaces.
The Need for Good Metrics
Even with a baseline of 3D imaging performance established, there is much to do to ensure accuracy and consistent results. Metrics are essential to ensuring that any 3D image capture system provides performance appropriate to the intended application. It is essential to quantify performance in ways that are meaningful and repeatable so that having the right specifications translates into reliable performance in the real world.
Known-good methods become even more valuable when they can point the way to potential improvements and enhancements of the 3D imaging solution. In addition to establishing a performance baseline, one is typically seeking to understand how robust a particular solution will be when challenged with diverse scenes, changes in perspective, and varying lighting conditions. The effect of each one of several possible conditions must be analyzed separately to provide a clear path to performance improvement.
Accuracy and Precision Metrics: Noise, Distortion, and Calibration Error
Different sources of error are mitigated using different methods. Let’s consider three aspects.
Noise: Precision of a depth measurement is largely set by the level of high-spatial-frequency fluctuations in the measured depth profile of a static object. Easily removed by a smoothing filter, such fluctuations are considered separately from other contributions.
Local distortion: Low spatial-frequency artifacts that do not look like noise may still distort the measured depth. These distortions cannot be easily filtered without compromising accuracy and spatial resolution. They may, for instance, deform a face’s surface profile beyond recognition despite high relative accuracy.
Calibration error: In addition to the two factors described already, other systematic errors may apply across the depth profile. These occur due to incorrect or incomplete calibration.
Whether or not the system is correctly calibrated, noise and local distortion largely determine the system’s depth resolution: the minimal depth required between two objects to confidently ascertain that they are not at the same distance from the sensor.
The noise, distortion, and calibration error fundamentally determine the accuracy of all depth measurements. In an ideal depth sensor, these three sources of error are absent, and the 3D point cloud data models the real-world scene with perfect fidelity.
Reproducibility Factors
Ideally, a depth sensor should perform consistently regardless of the content of the scene. A suitable quantitative test (fig. 2) of the consistency of same-depth measurements for non-equivalent target scenes is required. While standards for 3D depth measurement are an active topic of collaboration in the industry, it is possible to articulate this general concept. Certain features of a scene are most likely to degrade the reproducibility or fidelity of the data in the point cloud.
The most significant factors fall into the following six categories.
Color: Differently colored surfaces may be present in a scene illuminated by sunlight or artificial sources of white light. Furthermore, near-infrared illumination may be used in low lighting conditions. Regardless of the many contrasting colors that result, the depth profile of a surface should be reported accurately and consistently.
Contrast: As with stereo matching algorithms, AIRY3D’s DepthIQ™ technology uses edges to provide a sparse depth map. A wide range of contrasts in color, luminance and orientation are possible depending on the content of the scene. A robust 3D image capture technology should offer the same accuracy despite the complexity.
Range: The accuracy of depth estimation depends on the distance from the object to the camera. The loss of accuracy with depth determines the useful range of a particular 3D imaging solution in a given application.
Proximity: How much spatial separation must there be between two features if their depths are to be reported accurately? Some depth map post-processing algorithms can lead to changes in the measured profile of two features brought close by.
Motion: Any motion blur reduces contrast and sharpness of the image. The effect this has on depth measurement accuracy must be evaluated to determine the sensor’s usefulness in applications such as velocimetry.
Field of view: An ideal 3D imager would accurately report the depth of an object regardless of that object’s apparent position in the sensor’s
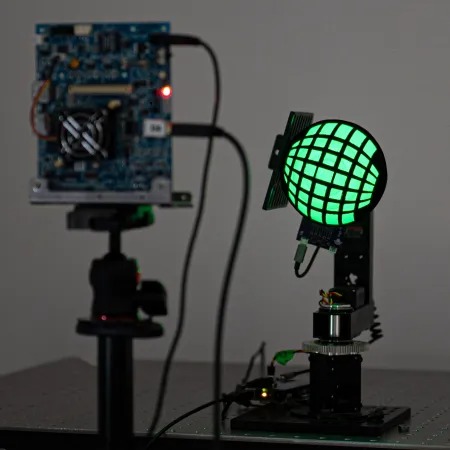
field of view.
A final word
Of course, vision industry experts from optical engineers to software developers will acknowledge that the short summary given above cannot fully encompass all the potential complexity of the many real-world situations addressed by 3D image capture technology.
Additional factors may need to be taken into consideration depending on your application, based on the tasks that your 3D imaging solution is designed to perform and the specific character of the targets, optics, and lighting. Moreover, the relevant scene parameters may significantly differ in the case of active solutions such as LIDAR or ToF sensors. This makes comparisons between the performance and robustness of different technologies quite challenging.
To this end, AIRY3D has joined others in the industry to develop meaningful standards for 3D/depth measurement accuracy across the whole technological landscape. The National Institute of Standards and Technology (NIST) in the US, together with standards body ASTM Committee E57, have released proceedings on a workshop, held late last year, to define standards for 3D imaging systems.
Standards are beneficial to both users and manufacturers. They allow manufacturers to determine specifications for their system and users to verify a system’s specifications under ideal or real-world conditions. Given the fast pace at which 3D perception solutions are developed and deployed, such efforts are poised to shape the future of these fascinating technologies.
AUTHORS: Félix Thouin, PhD, Imaging Scientist (AIRY3D); James Mihaychuk, Ph.D., PMC, Product Manager (AIRY3D)
For more information: www.airy3d.com
Tags: 3d vina, A Matter of Metrics – Key Metrics & Factors for Developing Robust 3D Imaging Solutions, hiệu chuẩn, hiệu chuẩn thiết bị, máy đo 2d, máy đo 3d, máy đo cmm, sửa máy đo 2d, sửa máy đo 3d, sửa máy đo cmm